Data Lineage
Data lineage is a concept that also has multiple ambiguous definitions. Several other concepts like data chain, integration architecture, data value chain, information value chain, and data flow have many things in common with the data lineage concept.
The key goal of data lineage is to trace data movement along data pipelines (chains) and to explain the transformations that data undergoes as it moves. There are three main challenges associated with data lineage documentation:
1. It can be done at various abstraction levels: business, conceptual/semantic, logical/solution, and physical.
2. Data objects at different levels should be linked to each other.
3. The term “data lineage” is misleading to some extent. Business leaders expect that they can trace data changes. If, for example, a financial report demonstrates revenue of 1 mln USD, financial professionals want to know all data changes and transformation that occurred back to underlying invoices. In reality, data lineage documents data processing by means of metadata. So, data lineage can explain movements and transformations from the origination data element “invoice” to the target data element “revenue” along a data chain. However, data lineage can’t explain what happened to a particular invoice along the data chains.
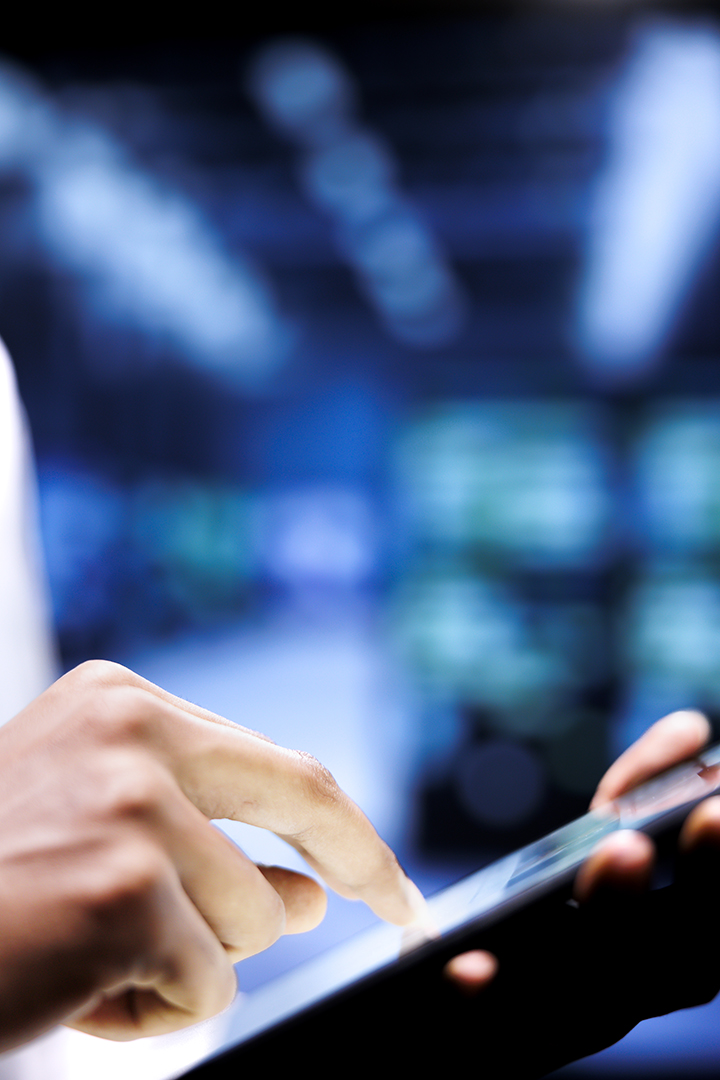
KNOWLEDGE GRAPHS AND DATA LINEAGE
WHY USE A KNOWLEDGE GRAPH FOR DATA LINEAGE?
The concepts of a knowledge graph and data lineage have a common goal: to retrieve and integrate metadata from different sources. Metadata can be stored in different repositories that include but are not limited to business glossaries, data models at conceptual/semantic levels, logical/solutions, physical levels, business processes, data dictionaries, data sets and IT catalogs, etc.
Sometimes the concept “data lineage” is taken to mean only the technical data lineage at the physical level that documents data movements in database tables and columns. Data lineage has a much broader scope than this.
While it may begin with a discrete data lineage initiative it often turns it into a knowledge graph project because compliance requires data lineage to be integrated with multiple systems of record.
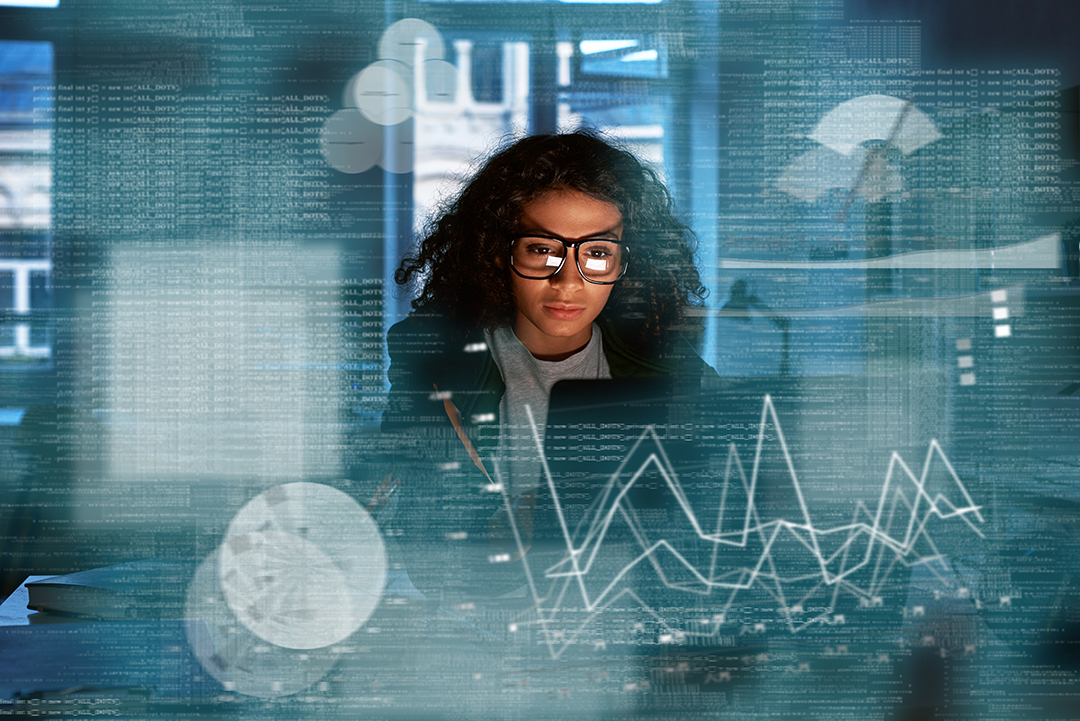
WHAT ARE THE KEY BENEFITS OF USING A KNOWLEDGE GRAPH FOR DATA LINEAGE?
Data lineage documentation can be done manually and automatically, depending on the level of abstraction. The documentation of data lineage at the business, conceptual/ semantic, and logical/solution levels can only be done manually. However, it is better that data lineage is documented at the physical level using automated solutions. Data lineage solutions must store and integrate metadata from many different existing applications, databases, and integration tools. Data lineage solutions combine repositories, visualization tools, and scanners to retrieve and ingest technical metadata into repositories.
Data lineage repositories can use both relational and graph databases. However, a graph database is one of the most effective advanced technologies that data lineage can use to reach its goals. In fact, a knowledge graph and data lineage in the context of data traceability and integration can be viewed as one concept.
A graph database technology eases the graphical representation of metadata objects and their relationships and information search capabilities.
Data lineage is metadata lineage that demonstrates the movement and transformation of data using metadata. Data graph technology offers a much broader ability: to link data and metadata. While feasible, such a solution can be very complex due to data and metadata volumes and (meta)data architecture and IT environments required for its realization.
WHAT SORTS OF COMPANIES NEED DATA LINEAGE AND KNOWLEDGE GRAPHS?
Two key reasons drive companies to implement data lineage and knowledge graphs: legislative requirements and business change.
Financial institutions worldwide must document data lineage because of the well-known “Principles for effective risk data aggregation and risk reporting” issued by the Basel Committee on Banking Supervision. Requirements for personal data protection are another reason to document data lineage.
Examples of business changes that require data lineage are digital transformation, change and optimization in the IT landscape and environment, and data management initiatives such as data quality.
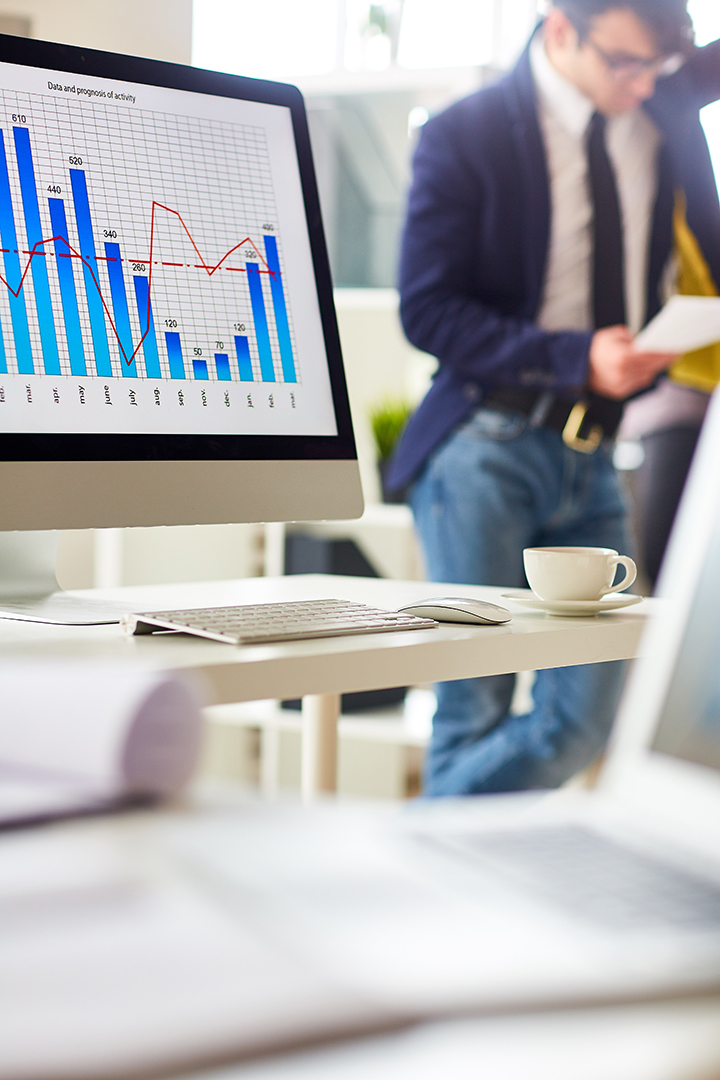
USE CASES
Data lineage/ knowledge graphs assist in performing many data management-related tasks. The key areas of applications are:
Explaining data origin and transformation
Internal and external audit and supervisory bodies approach a company’s board with requirements to explain the origin of figures that appear in the company’s reports. If a company does not have a documented data lineage/ knowledge graph in place, performing this task can take days, weeks, and even months. Data lineage streamlines this task significantly.
Performing impact and root-cause analysis
Many data management initiatives cannot be performed effectively without knowing the path of data movement and transformation across data pipelines. If a company starts a data quality initiative, it will need data lineage for root-cause and impact analysis. The root-cause analysis assists in explaining data quality issues and in building data quality checks. Another example is a change in source applications. Impact analysis assists in evaluating and planning changes required in data pipelines and reports.
Data lineage and knowledge graph initiatives interrelate with other business change and data management initiatives such as “digital transformation,” “customer-360 view,” “movement to a cloud,” and many others. Each of these initiatives is time- and resource-consuming but collectively they enable a company to remain competitive in the long-term.
NEXT STEPS
To learn more about how Trigyan can help you with your KYC project, click the button to contact us.